As the electronic age evolves and carries on to form the enterprise landscape, company networks have come to be significantly elaborate and dispersed. The total of details a corporation collects to detect malicious conduct frequently boosts, producing it challenging to detect deceptive and unidentified attack styles and the so-identified as “needle in the haystack”. With a increasing amount of cybersecurity threats, this sort of as facts breaches, ransomware attacks, and destructive insiders, organizations are struggling with substantial difficulties in productively monitoring and securing their networks. Furthermore, the expertise lack in the subject of cybersecurity can make handbook danger searching and log correlation a cumbersome and hard activity. To deal with these difficulties, organizations are turning to predictive analytics and Device Learning (ML) driven network security options as crucial equipment for securing their networks from cyber threats and the mysterious poor.
The Part of ML-Pushed Network Security Methods
ML-driven network security methods in cybersecurity refer to the use of self-mastering algorithms and other predictive systems (statistics, time examination, correlations and so forth.) to automate various facets of danger detection. The use of ML algorithms is getting to be progressively preferred for scalable systems because of to the limits current in classic rule-based mostly security options. This success in the processing of facts by means of innovative algorithms that can detect patterns, anomalies, and other subtle indicators of malicious action, such as new and evolving threats that may possibly not have identified terrible indicators or present signatures.
Detecting acknowledged danger indicators and blocking set up attack styles is continue to a essential portion of in general cyber hygiene. Even so, regular strategies utilizing threat feeds and static principles can turn into time-consuming when it comes to protecting and covering all the different log sources. In addition, Indicators of Attack (IoA) or Indicators of Compromise (IoC) may well not be offered at the time of an attack or are promptly out-of-date. Consequently, corporations need other methods to fill this gap in their cybersecurity posture.
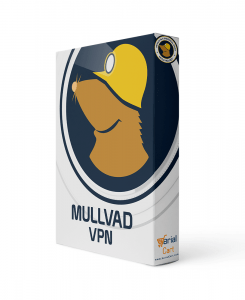
Protect your privacy by Mullvad VPN. Mullvad VPN is one of the famous brands in the security and privacy world. With Mullvad VPN you will not even be asked for your email address. No log policy, no data from you will be saved. Get your license key now from the official distributor of Mullvad with discount: SerialCart® (Limited Offer).
➤ Get Mullvad VPN with 12% Discount
In summary, the mentioned drawbacks of rule-primarily based security methods emphasize the importance of using a much more holistic approach to network security, which must nowadays involve ML-powered Network Detection and Reaction (NDR) alternatives to enhance standard detection capabilities and preventive security measures.
The Benefits of ML for Network Security
So, how is Device Mastering (ML) shaping the potential of network security? The real truth is ML-powered security options are bringing about a important transformation in network security by delivering security groups with several rewards and boosting the total menace detection capabilities of corporations:
- Significant knowledge analytics:With the at any time-escalating sum of information and unique log resources, organisations need to be ready to system vast quantities of facts in true-time, including network targeted visitors logs, endpoints, and other sources of data connected to cyber threats. In this regard, ML algorithms can assist in the detection of security threats by determining styles and anomalies that may well in any other case go unnoticed. For that reason, the capacity and overall flexibility of a resolution to include various log resources should be a key necessity for threat detection abilities.
- Automatic assessment of anomalous conduct: AI enables a considerably-expected wellbeing checking of network action by utilising the analysis of standard network targeted traffic as a baseline. With the assistance of automatic correlation and clustering, outliers and abnormal actions can be detected, reducing the need for handbook detection engineering and risk looking. Key inquiries to be answered involve “what is the exercise of other shoppers in the network?” and “is a client’s conduct in line with its possess previous routines?” These methods make it possible for for the detection of unusual behaviors like domain-created algorithms (DGA) domains, volume-dependent irregularities in network connections, and strange conversation designs (e.g., lateral motion) in the network. Consequently, evaluating a client’s present habits with that of its friends serves as a appropriate baseline for pinpointing refined anomalies.
- Detect mysterious attacks in real-time: Whileit is rather effortless to right detect recognised bad indicators (distinct IP addresses, domains and so forth.), a lot of attacks can go undetected when these indicators are not current. If that is the case, figures, time and correlation-primarily based detections are of massive value to detect mysterious attack styles in an automated fashion. By incorporating algorithmic approaches, conventional security answers based on signatures and indicators of compromise (IoC) can be improved to turn into extra self-adequate and less reliant on recognised malware indicators.
- Self-discovering detection abilities: ML-pushed answers discover from past situations in get to continually boost their risk detection abilities, menace scoring, clustering and network visualisations. This might involve schooling the algorithms them selves or changing how information is introduced primarily based on opinions from analysts.
- Enhance Incident Reaction:By learning from an analyst’s past incident response functions, ML can automate specific elements of the incident response approach, minimizing the time and sources necessary to deal with a security breach. This can involve employing algorithms to examine text and proof, pinpointing root results in and attack patterns.
Example of an ML-pushed Network Security Solution
When it will come to ML-driven Network Detection & Response (NDR) methods that integrate the outlined gains, ExeonTrace stands out as a top network security answer in Europe. Primarily based on award-profitable ML algorithms, which incorporate a decade of tutorial investigate, ExeonTrace presents organizations with highly developed ML threat detection abilities, full network visibility, versatile log source integration and significant facts analytics. In addition, the algorithms rely on metadata analysis instead of true payloads which would make them unaffected by encryption, wholly components-absolutely free and appropriate with most cybersecurity infrastructures. As a outcome, ExeonTrace is ready to procedure raw log data into strong graph databases, which are then analyzed by supervised and unsupervised ML-products. As a result of correlation and occasion fusion, the algorithms can precisely pinpoint superior-fidelity anomalies and subtle cues of malicious behavior, even when dealing with novel or emerging cyber threats that could absence recognized signatures or regarded malicious indicators.
Security Analytics Pipeline: Detection of network anomalies as a result of ML
Conclusion
As the danger of cyber attacks gets to be progressively intricate, corporations need to go further than classic security measures to defend their networks. As a result, a lot of businesses are now turning to Device Understanding (ML) and predictive analytics to reinforce their security defenses. In this regard, ML-pushed Network Detection & Reaction (NDR) remedies, such as ExeonTrace, are built to aid organizations keep ahead of the ever-evolving danger landscape. By utilizing superior ML algorithms that review network traffic and application logs, ExeonTrace offers corporations quick detection and response to even the most sophisticated cyberattacks.
ExeonTrace Platform: Network visibility
Book a free demo to learn how ExeonTrace leverages ML algorithms to make your organisation a lot more cyber resilient – quickly, responsible and wholly components-free.
Uncovered this short article attention-grabbing? Stick to us on Twitter and LinkedIn to study much more special material we article.
Some components of this post are sourced from:
thehackernews.com